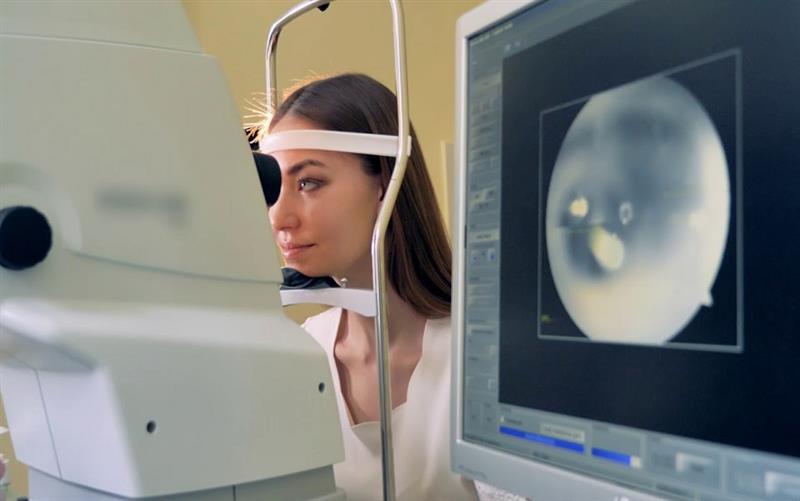
Ophthalmologists won't be replaced by AI, but those that use it well will probably establish the benchmark for eye care in the future. We can anticipate fewer diagnostic errors, better results, and increased access to high-quality eye care on a worldwide scale as software develops further and becomes more smoothly integrated into clinical practice.
Ophthalmology occupies a special place at the nexus of precision and technology in the rapidly changing healthcare industry of today. The margin for error is extremely narrow because millions of patients depend on prompt and precise diagnosis of eye disorders, ranging from age-related macular degeneration to glaucoma and diabetic retinopathy. However, inaccurate diagnoses continue to be a problem, frequently resulting in postponed treatments, avoidable vision loss, and higher medical expenses.
Here comes ophthalmology software driven by AI. AI is quickly changing eye care into a more precise, effective, and accessible field by fusing the enormous datasets produced by contemporary imaging technologies with the diagnostic capabilities of machine learning.
The Range of Ophthalmology Diagnostic Errors
In ophthalmology, diagnostic errors are not unusual. They have several root causes, such as:
Image interpretation that is subjective
Clinicians who are overworked and dealing with a lot of patients
Differences in experience and training
Limitations of established approaches for early-stage detection
These mistakes may lead to misclassifications or missed diagnoses, which may affect treatment plans and results. Early identification is essential for conditions like glaucoma, and even a few months of delay can cause irreparable harm.
Key Benefits:
1. Enhanced Diagnostic Accuracy
Manually interpreting retinal pictures or OCT (Optical Coherence Tomography) scans is a common practice in traditional eye diagnosis. Despite their great skill, human clinicians can still make mistakes, particularly when abnormalities are subtle or unclear.
AI algorithms that have been trained on enormous collections of tagged ophthalmic photos are able to identify intricate and early symptoms of illnesses such as
Diabetic retinopathy, including hemorrhages and microaneurysms
Glaucoma (thinning of the RNFL, cupping of the optic nerve)
Macular degeneration associated with aging (AMD) (drusen, pigmentary alterations)
Deep learning is used by these algorithms to find patterns and abnormalities that might not be immediately apparent to the human eye, particularly in the early phases. For instance, research has demonstrated that AI models can diagnose some illnesses with diagnostic sensitivity and specificity that are on par with or better than those of qualified ophthalmologists.
2. Consistency Across Populations
Human interpretation is inherently diverse. A case of diabetic retinopathy may be classified as mild by one clinician and as moderate by another. Results may be impacted by elements such as mood, experience level, or even weariness.
AI consistently produces diagnostic results regardless of:
Who is making use of it?
Where it's utilized
When it's utilized
AI models consistently apply the same criteria to every image after they have been trained and validated. This lessens subjectivity and promotes uniform care, which is particularly beneficial in screening programs, multicenter networks, and situations when obtaining second opinions is challenging.
3. Speed and Scalability
It takes a lot of time to manually evaluate ophthalmic photographs, particularly in high-volume settings like community eye camps or diabetic retinopathy screening programs. In a fraction of the time required by a human expert, AI software can process hundreds of photos.
Here, the main benefits are:
Real-time screening: Immediate evaluation while the patient remains in the room
Bulk processing: Reviewing images in bulk for studies or big populations
Automated triage: Giving urgent care patients priority
4. Clinical Decision Support
AI generates more than just binary results like "disease present" or "disease absent." Numerous systems have characteristics that aid in decision-making, including:
Probability scores, such as the 92% chance of macular edema
Region-based highlights or heatmaps
Recommended diagnosis or actions to take next
EHR integration for documentation and alerts
This helps clinicians verify data or think of differential diagnoses they would not have previously considered, giving them greater context and confidence in their diagnostic process. Additionally, it can help general practitioners or early career professionals who do not have an on-site ophthalmologist.
5. Early Detection and Preventive Care
The potential of AI to identify illness signs before they become clinically visible is one of its most potent features. AI can accurately identify, for example, early microaneurysms in diabetic retinopathy or small alterations in the layers of retinal nerve fibers in glaucoma. Clinicians can greatly lower the chance of irreparable damage by identifying these symptoms early, starting preventive medications, keeping a closer eye on patients, and stopping the progression of the disease.
This makes prevention the primary line of defense and changes the clinical strategy from reactive to proactive.
6. Improved Access in Remote and Underserved Areas
The lack of specialists in rural and underdeveloped areas is a major obstacle to global eye care. Handheld fundus cameras, smartphone apps, and cloud-based platforms that do not require an in-person ophthalmologist can all incorporate AI-powered diagnostic tools.
For instance, AI can take retinal scans and provide immediate diagnostic input to general practitioners or community health workers. This enables prompt referrals and the reduction of health inequities globally by enabling the delivery of specialist-level tests to remote places.
7. Continuous Learning and Improvement
AI systems are dynamic, in contrast to conventional diagnostic instruments. They get better over time as they are exposed to new data, particularly from rare disorders and different populations. Federated learning methods, which are used by many platforms, enable AI to learn from data from several centers without jeopardizing patient privacy.
The diagnosis process is kept current, flexible, and pertinent by AI tools' ability to adapt to changing clinical standards, diagnostic trends, and even changes in illness presentation.
8. Reduced Clinician Burnout
The repetitious picture analysis that ophthalmologists frequently perform can be cognitively exhausting and prone to human mistake, particularly when under pressure. AI lessens this load by:
Automating preliminary screenings
Giving high-risk cases priority
Highlighting pictures that need more consideration
This ultimately results in less stress, better focus, and more job satisfaction by enabling physicians to devote more time to complex situations, interact more intimately with patients, and make better decisions.
9. Cost-Efficiency Over Time
AI streamlines the diagnostic procedure, which lowers healthcare costs:
There are fewer follow-up appointments and needless treatments when there are fewer misdiagnoses.
Automated triage can save money on staffing and lessen the workload for professionals.
Only patients with urgent problems are given priority for in-person appointments thanks to better resource allocation.
The long-term return on investment (ROI)—in the form of improved results, increased operational efficiency, and less liability—makes AI a cost-effective solution for both large institutions and private practices, even though initial implementation may involve investments in hardware, software, and training.
10. Enhanced Patient Engagement and Transparency
Visual outputs from AI systems, such as color-coded heatmaps that highlight problematic areas in a picture, are frequently included. Patients gain a better understanding of their illness when these images are shown to them. Instead of using intricate medical terminology, AI-assisted consultations can consist of:
Basic justifications for the results
Comparisons with earlier pictures
Risk ratings for the advancement of the disease
People are empowered to take a more active role in maintaining their eye health when there is this degree of openness and visual communication, which also promotes patient trust and treatment plan adherence.